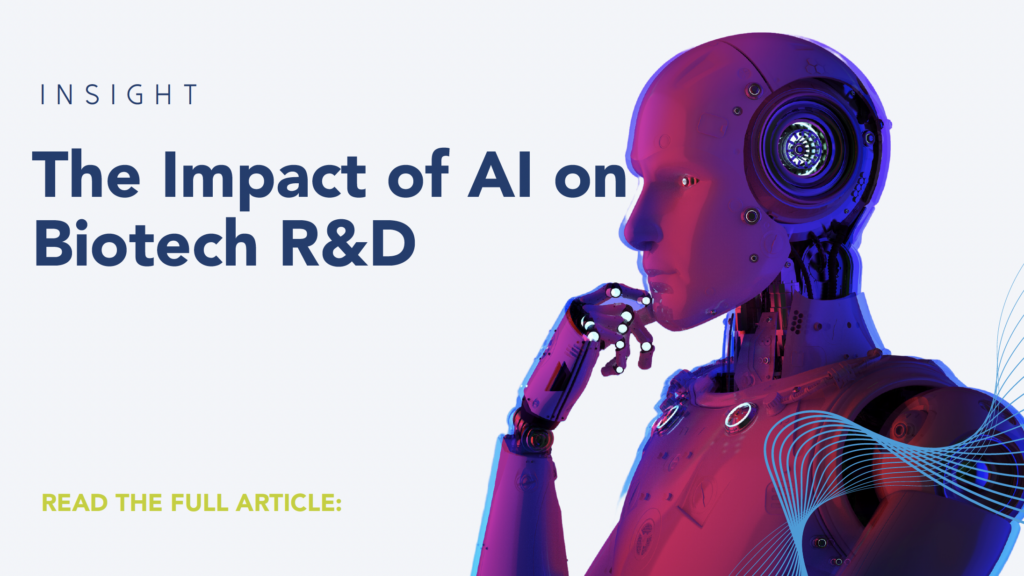
The use of AI in biotech research and development has the potential to revolutionise the way we discover, develop and manufacture biologics. With the growth of large datasets and increased computational power, AI has become an essential tool in the biotech industry.
The field of drug discovery in biotech R&D is undergoing a significant transformation with the application of AI. Advanced AI algorithms are capable of analysing vast amounts of data, ranging from genomic and clinical data to scientific literature, to identify new drug targets and refine existing drug candidates. By leveraging AI, the drug discovery process can be significantly accelerated, while also improving the success rate of drug development.
Impressive results have already been demonstrated through AI based drug discovery, with several examples of novel drug candidates identified through AI-powered analysis. Insilico Medicine recently used its AI platform to identify and validate potential drug targets for idiopathic pulmonary fibrosis. Similarly, Exscientia’s AI based platform identified a drug candidate for Obsessive-Compulsive Disorder (OCD) that entered Phase 1 clinical trials in record time. As AI continues to evolve and improve, its impact on biotech research and development is expected to become even more significant. The use of AI in drug discovery has the potential to transform the entire pharmaceutical industry, bringing about faster, more efficient drug development and ultimately improving patient outcomes.
AI is also proving to be a game-changer in bioprocess optimisation. This involves improving the efficiency and productivity of bioprocessing operations such as cell culture, fermentation, purification, and formulation. Optimisation can help reduce costs and increase product quality, as well as scale up production for commercialisation. AI algorithms can analyse real-time data from various sensors and monitoring equipment in bioprocessing operations. With this information, AI can predict how different parameters will affect the performance of the bioprocess. The bioprocess can then be further optimised to improve yields, reduce costs, and increase efficiency.
For example, an AI system can analyse the data generated from bioprocess sensors and predict the optimal time to harvest cells or perform a downstream purification step. This can help reduce the time and resources required for bioprocessing, as well as minimise the risk of contamination or product loss. Furthermore, AI can enable biotech companies to scale up their production processes more efficiently. By optimising bioprocess parameters and identifying bottlenecks in production, AI can help identify areas where process improvements are needed. This can enable companies to quickly and efficiently increase their production capacity and meet the growing demand for biotech products.
AI can also help to reduce the time and cost of clinical trials by improving patient recruitment and identifying more targeted patient populations. AI-powered clinical trial management systems can analyse patient data to identify the most suitable candidates for clinical trials, as well as monitor patient health in real-time to ensure patient safety and improve trial outcomes.
However, there are also challenges associated with the use of AI in biotech research and development. One of the biggest challenges is the need for high-quality, standardised data to feed into AI algorithms. This requires collaboration between different stakeholders in the industry, as well as the development of robust data sharing platforms. As AI algorithms become increasingly complex and powerful, there is a need for robust regulations to ensure that AI-powered products are safe and effective for patients.
AI has the potential to transform biotech research and development by accelerating drug discovery, optimising bioprocesses, and improving clinical trial outcomes. However, there are also challenges associated with the use of AI in biotech, and these must be addressed through collaboration and the development of robust regulatory frameworks.
References:
- Gallo Cassarino, T. et al. (2020). Artificial intelligence in biotech research and development: current status and future prospects. Expert Opinion on Drug Discovery, 15(11), 1303-1315.
- Hao, L. & Wang, J. (2021). AI for biotechnology and biopharmaceuticals. Trends in Biotechnology, 39(7), 710-723.
- Cai, X. et al. (2021). AI-assisted bioprocessing: Recent advances and future outlooks. Current Opinion in Biotechnology, 70, 95-104.
- Smalley, E. (2021). The role of artificial intelligence in biopharmaceutical research and development. Drug Discovery Today, 26(3), 708-716.
- Sliwoski, G. et al. (2021). Advancing Drug Discovery with Artificial Intelligence: A Perspective on the State of the Art. Drug Discovery Today, 26(4), 707-715.